Breakthrough: Large Language Models Pass the Turing Test
For over 70 years, the Turing Test has stood as the quintessential benchmark for artificial intelligence. Proposed by Alan Turing in 1950, it evaluates a machine's ability to exhibit intelligent behavior indistinguishable from that of a human. Now, in a groundbreaking development, researchers have demonstrated that advanced Large Language Models (LLMs) can successfully pass this legendary test, marking a significant milestone in AI development.
The Historic Achievement
A recent study conducted by researchers from UC San Diego has provided empirical evidence that modern LLMs can successfully imitate human conversation to the point where they are indistinguishable from, or even preferred over, actual humans [1]. In their experiment, participants engaged in 5-minute conversations simultaneously with both a human and an AI before judging which conversational partner they thought was human.
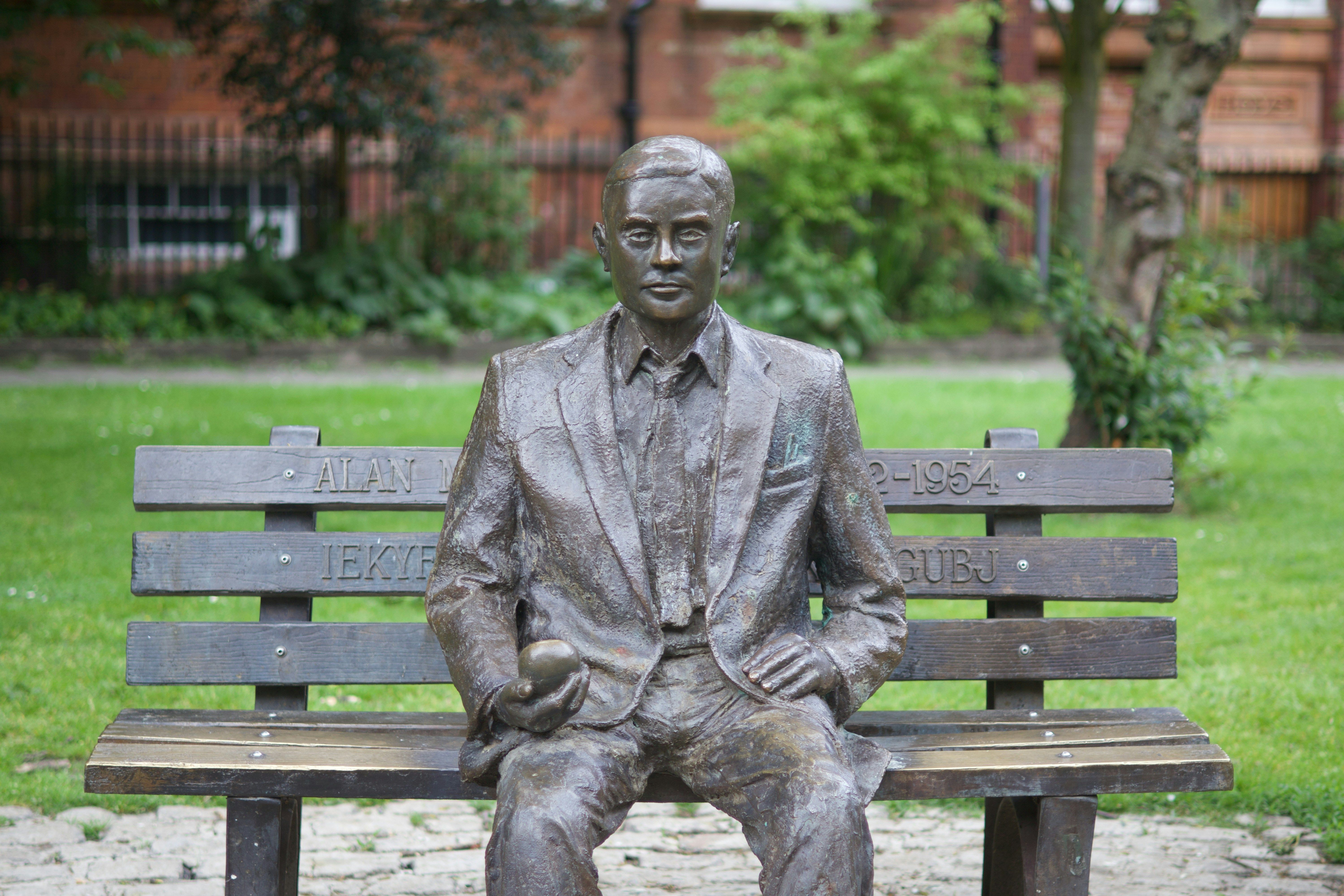
The results were remarkable: when prompted to adopt a humanlike persona, OpenAI's GPT-4.5 was judged to be human 73% of the time — significantly more often than participants selected the actual human [1]. Meta's LLaMa-3.1-405B model achieved a 56% success rate, essentially matching human performance. Meanwhile, baseline models like ELIZA and GPT-4o were correctly identified as non-human, achieving success rates of only 23% and 21% respectively.
“People were no better than chance at distinguishing humans from GPT-4.5 and LLaMa (with the persona prompt).”
— Cameron Jones, Lead Researcher, UC San Diego's Language and Cognition Lab
Understanding the Implications
While this achievement represents a significant technical milestone, researchers caution that passing the Turing Test doesn't necessarily settle the debate about machine intelligence. Instead, it provides one data point among many for evaluating the capabilities of modern LLMs.
Technical Perspective
The advancement highlights the remarkable progress in language model development. These systems can now process context, generate coherent responses, and adapt their communication style to appear more human-like with appropriate prompting [2].
Societal Implications
This capability raises important questions about the potential for AI to substitute for humans in certain interactions without detection, with implications for job automation, cybersecurity, and broader social dynamics [3].
The Reverse Turing Test Phenomenon
Interestingly, as LLMs become more sophisticated, researchers have identified what they call the "Reverse Turing Test" phenomenon. This concept suggests that what appears to be intelligence in LLMs may actually be a mirror reflecting the intelligence of the human interviewer [4].
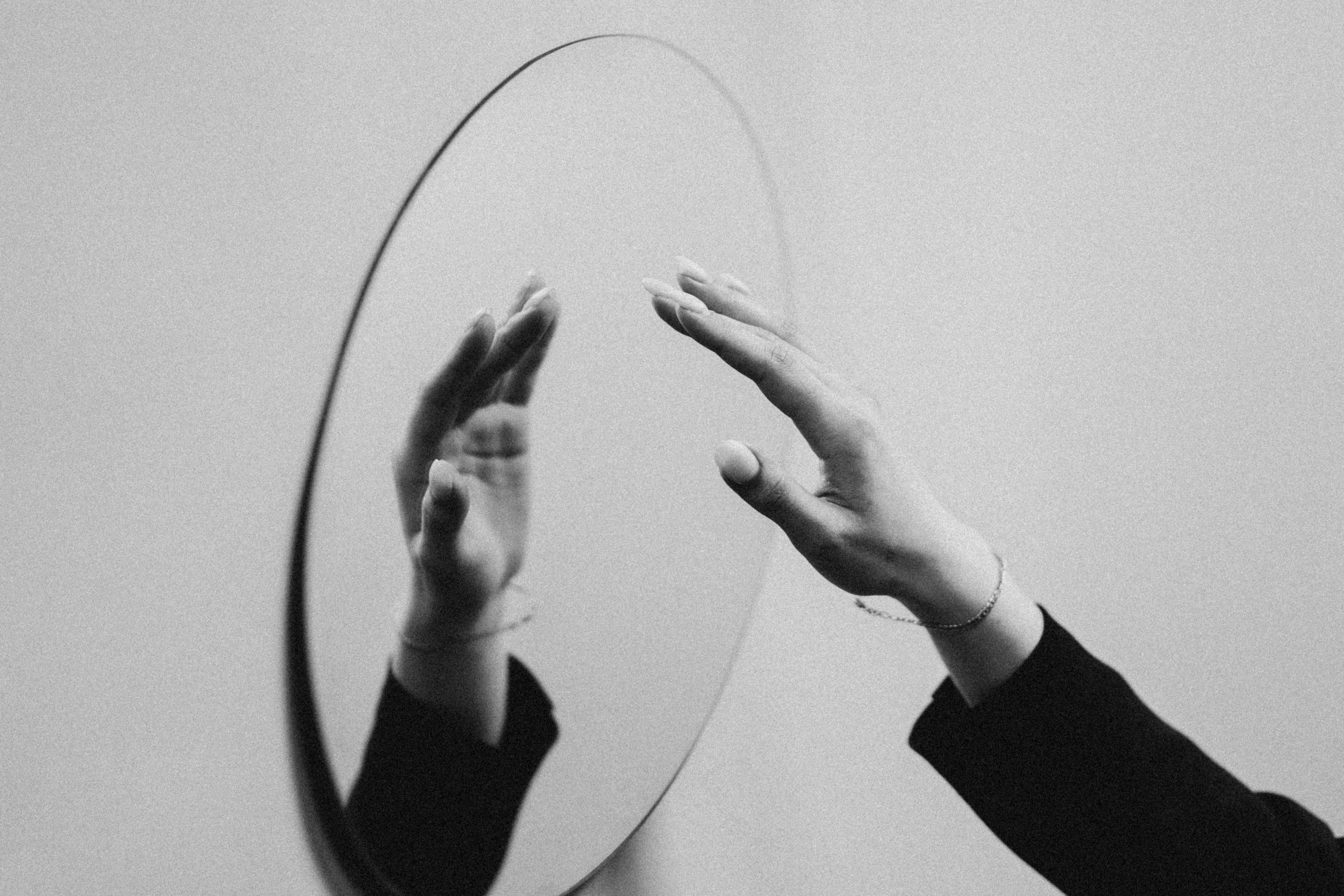
The Mirror Effect
When interacting with advanced LLMs, the quality and intelligence of the conversation may depend significantly on the human's input, creating what researchers term a "remarkable twist" in how we evaluate machine intelligence [4]. This suggests that when we study interactions with AI systems, we may be learning as much about human intelligence as we are about artificial intelligence.
Challenging the Value of the Test
Some researchers and observers have raised valid critiques about the methodology and significance of modern Turing Test experiments. One critique noted in community discussions suggests that participants in the UC San Diego study might not have made significant efforts to unmask the AI, potentially limiting the robustness of the findings [5].
Additionally, as the public becomes more familiar with AI interactions, their ability to detect artificial conversation partners may improve over time. This suggests that passing the Turing Test may be a moving target rather than a fixed milestone.
Beyond Language: The Next Frontier
While achieving human-like conversational abilities represents a significant accomplishment, researchers recognize this as just one aspect of intelligence. The next challenge involves integrating language capabilities with sensorimotor functions and decision-making systems.
From Talk to Action
As one researcher aptly phrased it, "LLMs can talk the talk, but can they walk the walk?" [4] This question highlights the next frontier in AI development: creating systems that can not only communicate effectively but also understand and interact with the physical world in meaningful ways.
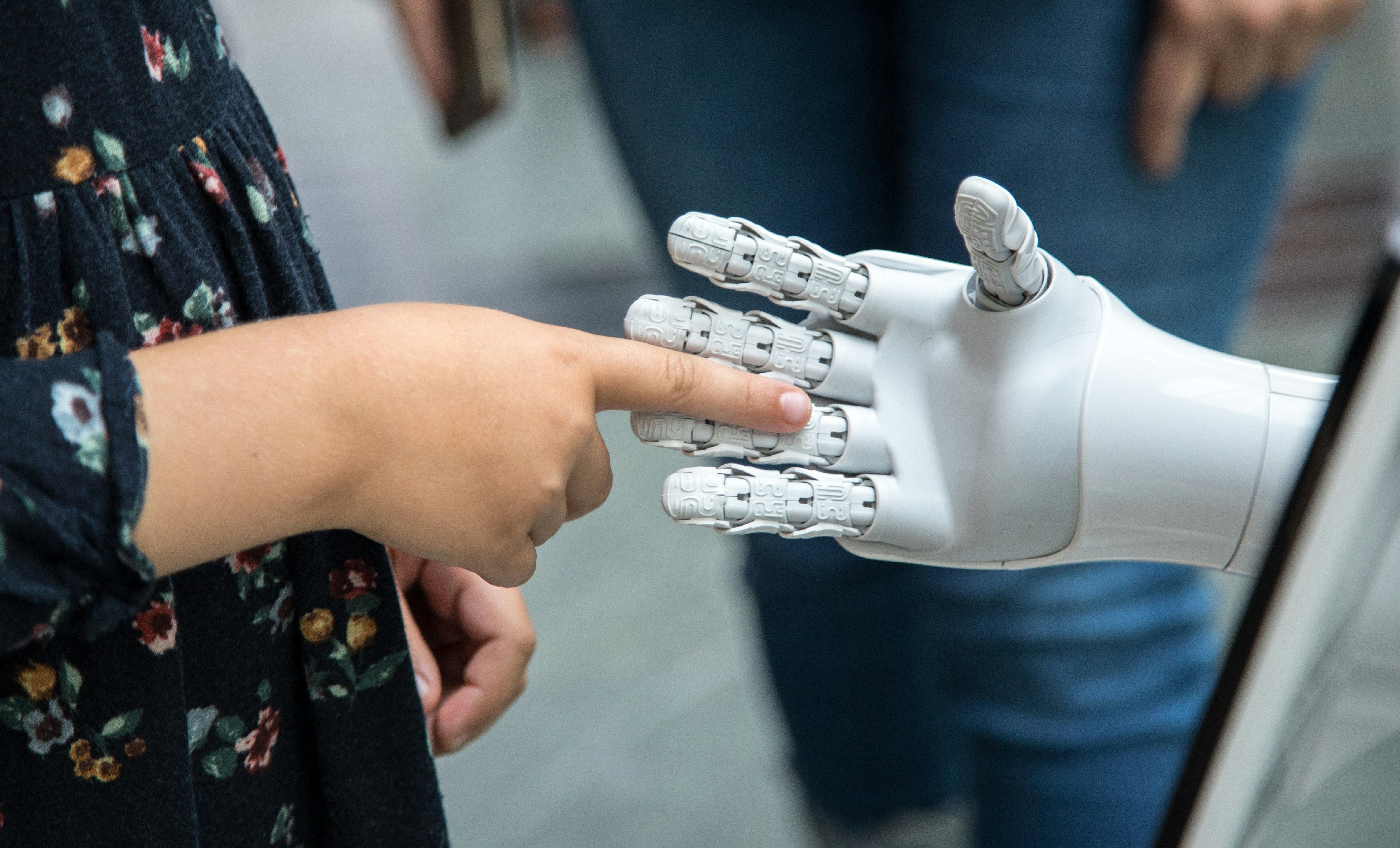
Looking Forward
The achievement of passing the Turing Test marks a significant milestone in AI development, but researchers emphasize that it represents the beginning of a new chapter rather than the conclusion of the AI story. Many compare current LLMs to the Wright brothers' first flight — a proof of concept that demonstrates possibility while highlighting how much further development lies ahead [2].
As research continues to advance, future developments will likely focus on integrating language capabilities with other cognitive functions, addressing current limitations like hallucinations, and developing more robust frameworks for evaluating different dimensions of machine intelligence.
A New Era of Human-AI Interaction
With LLMs now capable of passing as human in conversational settings, we're entering a new era where the boundaries between human and machine communication become increasingly blurred. This transformation will undoubtedly reshape how we interact with technology, demanding new frameworks for understanding, evaluating, and leveraging artificial intelligence in responsible and beneficial ways.
References
1 C. R. Jones and B. K. Bergen, "Large Language Models Pass the Turing Test," arXiv, 2503.23674, 2025, Online.
2 T. J. Sejnowski, "Large Language Models and the Reverse Turing Test," Neural Computation, vol. 35, no. 3, pp. 309-320, 2023, Online.
3 J. Davies, "An AI Model Has Officially Passed the Turing Test," Futurism, 2025, Online.
4 T. J. Sejnowski, "Large Language Models and the Reverse Turing Test," arXiv, 2207.14382, 2022, Online.
5 Anonymous, "UCSD: Large Language Models Pass the Turing Test," Hacker News, 2025, Online.
Transforming Legal Practices: The Revolutionary Role of AI in Document Analysis
Explore how artificial intelligence is reshaping legal document analysis, enhancing efficiency, accuracy, and cost-effectiveness in the legal profession. This article delves into key technologies driving change and future implications for legal practices.
The Evolving Role of Software Engineers in the Era of Vibe Coding
An in-depth analysis of how vibe coding is transforming software engineering practices, examining its impact on professional roles, team structures, and development methodologies. This research explores both opportunities and challenges in this AI-driven paradigm shift.