Specialized RAG Systems in Healthcare: Transforming Clinical Decision-Making Through Intelligent Data Integration
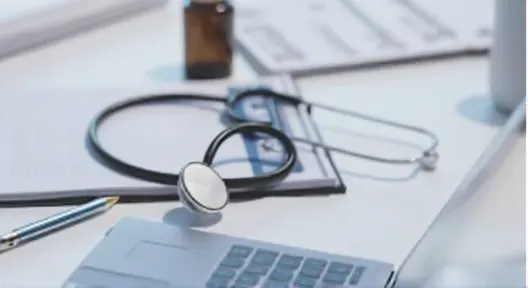
In today's complex healthcare landscape, clinicians face an overwhelming deluge of medical information that must be processed and integrated for optimal patient care. The emergence of Retrieval-Augmented Generation (RAG) systems represents a significant breakthrough in how healthcare providers can synthesize vast amounts of medical research, patient data, and treatment protocols to make more informed clinical decisions. This comprehensive exploration examines how specialized RAG systems are revolutionizing healthcare decision support by intelligently integrating diverse data sources, enhancing diagnostic accuracy, and enabling truly personalized treatment planning.
The Evolution of Clinical Decision Support and the RAG Revolution
Healthcare decision-making has traditionally relied on clinicians' ability to manually integrate information from disparate sources including medical literature, patient records, and clinical guidelines. This process has been inherently limited by human cognitive capacity, time constraints, and the exponential growth of medical knowledge. The introduction of early clinical decision support systems offered modest improvements but often suffered from outdated information and limited intelligence (Smart Data Integration).
Retrieval-Augmented Generation represents a paradigm shift in clinical decision support by combining the reasoning capabilities of large language models (LLMs) with the ability to dynamically retrieve and incorporate external knowledge. Unlike traditional AI models that rely solely on pre-trained knowledge, RAG systems can access up-to-date medical literature, clinical guidelines, and patient-specific information to ground their outputs in factual, relevant data (Nature). This approach significantly reduces the risk of hallucinations (factually incorrect information) and enhances the reliability of AI recommendations in clinical settings.
The core innovation of RAG lies in its hybrid architecture that separates knowledge retrieval from generation. When presented with a clinical query, the system first identifies and retrieves relevant information from validated knowledge sources, then uses this retrieved context to generate accurate, contextually appropriate responses. This architecture ensures that recommendations reflect the latest medical evidence while maintaining the sophisticated reasoning capabilities of advanced language models (Thiyagarajan).
Architectural Innovations in Healthcare RAG Systems
Modern healthcare RAG systems employ sophisticated architectures designed specifically for the complexities of medical decision-making. One notable innovation is the hierarchical RAG architecture exemplified by systems like HiRMed (Hierarchical RAG-enhanced Medical Test Recommendation). This approach implements a tree-structured design where each node incorporates specialized RAG processes, enabling progressive refinement of recommendations through multiple levels of medical reasoning (Yang & Huang).
These hierarchical systems typically employ a three-layer architecture: a root layer for initial analysis and department routing, a department layer for specialized knowledge retrieval, and an item layer for final test recommendations. This structure mirrors the real-world diagnostic process, beginning with broad assessment and progressively narrowing to specific interventions. The multi-layered approach, combined with dedicated memory components and weighting mechanisms, provides an adaptive framework that accommodates the complexity of clinical scenarios while maintaining transparent reasoning (Yang & Huang).
Another architectural advancement is the incorporation of knowledge graphs into RAG frameworks. MedRAG, for instance, systematically constructs a four-tier hierarchical diagnostic knowledge graph encompassing critical differences between various diseases. This comprehensive organization allows for enhanced precision in disease differentiation and diagnosis, enabling better decision-making support for conditions with similar presentations (MedRAG). By dynamically integrating these knowledge structures with similar electronic health records retrieved from databases, such systems can reason more effectively within large language models.
Clinical Applications and Performance Benchmarks
Healthcare RAG systems have demonstrated remarkable effectiveness across multiple clinical domains. In preoperative medicine, RAG-enhanced models have achieved 91.4% accuracy in generating appropriate recommendations, significantly outperforming both basic language models (80.1%) and human junior doctors (86.3%). These systems generate answers within 15-20 seconds, compared to the typical 10 minutes required by humans, representing substantial efficiency gains in time-critical clinical environments (Development and Testing).
Oncology represents another area where RAG systems are making significant impacts. Innovative approaches like Agentic-RAG and Graph-RAG have been developed to generate cancer treatment recommendations based on National Comprehensive Cancer Network (NCCN) guidelines. In evaluation studies, Agentic-RAG achieved 100% adherence with no hallucinations or incorrect treatments, while Graph-RAG demonstrated 95.8% adherence. Both systems provided detailed treatment recommendations with accurate references to relevant guideline documents and page numbers, enhancing their clinical credibility (Artificial Intelligence Tool).
Medication safety has also benefited from RAG implementation. Clinical trials have shown that RAG-LLM based clinical decision support systems significantly boost the accuracy of medication error identification when used alongside junior pharmacists. These systems excel in detecting moderate to severe drug-related problems, potentially preventing harmful medication errors before they reach patients (Novel Large Language Model-Based).
Enhancing Precision Medicine Through Personalized Data Integration
One of the most promising applications of healthcare RAG systems lies in advancing precision medicine initiatives. Traditional AI models typically provide similar general clinical advice to patients exhibiting similar symptoms, but RAG systems can comprehensively analyze a patient's biomarkers, genetic profiles, and molecular data to classify them into more granular subgroups and recommend personalized treatment plans (Nature).
By retrieving and integrating a patient's complex clinical and molecular data, RAG systems empower physicians to develop more accurate and personalized treatment plans tailored to each individual. For example, while two cancer patients may present with similar symptoms, their disease progression and prognoses may differ significantly due to variations in their biomarkers. RAG systems can leverage this detailed information to recommend more precise interventions (Nature).
The personalization capabilities extend beyond treatment recommendations to preventive care as well. For individuals with identified genetic risk factors, such as predisposition to heart disease, RAG systems can analyze personal health data, lifestyle factors, environmental conditions, and genetic information to recommend specific dietary plans and exercise regimens tailored to reduce their particular risk profile. This level of customization represents a significant advancement over generic health recommendations (MyScale).
Challenges and Ethical Considerations in Medical RAG Implementation
Despite their promising capabilities, healthcare RAG systems face significant implementation challenges. Data privacy represents a paramount concern, particularly given the sensitivity of medical information. These systems must integrate private and personal information while maintaining robust security measures to prevent unauthorized access or data breaches (Retrieval Augmented Thought Process).
Scalability presents another critical challenge as medical data continues to grow at a compound annual growth rate exceeding 35%. RAG systems must efficiently process and retrieve relevant information from this expanding data landscape without compromising speed or accuracy, particularly in real-time clinical applications where timely access to information directly impacts patient outcomes (NVIDIA).
Bias mitigation remains an ongoing concern in healthcare AI. While RAG systems can potentially reduce some forms of bias by retrieving information specific to underrepresented populations, the retrieval process itself may introduce new biases if the external knowledge sources contain skewed or limited information about certain demographic groups. The lack of high-quality information on underrepresented groups means minority populations may benefit less from existing RAG implementations (Nature).
Transparency and interpretability challenges also persist. Although RAG systems can enhance transparency by providing evidence for their recommendations, determining which specific retrieved documents influenced particular parts of a response remains difficult without human inspection. This challenge is compounded when knowledge conflicts exist between different retrieved sources or between retrieved information and the model's internal knowledge (Nature).
Future Directions and Integration Pathways
The future of RAG in healthcare will likely involve deeper integration with existing clinical workflows and electronic health record (EHR) systems. Successfully embedding these technologies into everyday clinical practice requires addressing interoperability challenges to ensure seamless data exchange between RAG systems and other healthcare IT infrastructure (Nature).
Multimodal RAG systems represent another promising frontier, incorporating not just text but also medical imaging, sensor data, and other information types. Hybrid RAG-empowered medical Multi-modal Large Language Models (MLLMs) are being developed to manage diverse healthcare data formats, potentially enabling more comprehensive analysis and decision support (Hybrid RAG-empowered).
Emotional intelligence is emerging as an unexpected enhancement to clinical RAG systems. Some innovative approaches incorporate sentiment analysis to detect and respond to the emotional state of healthcare providers using the system. By adapting communication style and content based on the clinician's emotional state, these systems aim to reduce cognitive load and provide empathetic support in high-stress clinical environments (AXAI-CDSS).
Conclusion
Specialized RAG systems represent a transformative development in healthcare decision support, offering unprecedented capabilities to synthesize diverse medical information and translate it into actionable clinical insights. By intelligently integrating medical research, patient data, and treatment protocols, these systems are enabling more informed, personalized, and evidence-based clinical decision-making.
While significant challenges remain in terms of privacy protection, bias mitigation, and clinical integration, the potential benefits for patient care are substantial. As these technologies continue to evolve through multidisciplinary collaboration among clinicians, researchers, and technologists, they promise to become invaluable partners in clinical practice—augmenting rather than replacing human expertise and ultimately improving patient outcomes through more precise, personalized, and timely care decisions.
References
1 Nature, Retrieval-augmented generation for generative artificial intelligence in healthcare, (Nature)https://www.nature.com/articles/s44401-024-00004-1
2 Arxiv, Development and Testing of Retrieval Augmented Generation in Large Language Models, (Arxiv)https://arxiv.org/abs/2402.01733
3 Arxiv, Developing an Artificial Intelligence Tool for Personalized Breast Cancer Treatment Plans based on the NCCN Guidelines, (Arxiv)https://arxiv.org/html/2502.15698v1
4 Arxiv, AXAI-CDSS: An Affective Explainable AI-Driven Clinical Decision Support System, (Arxiv)https://arxiv.org/html/2503.06463v1
5 Yang & Huang, A Tree-based RAG-Agent Recommendation System, (Arxiv)https://arxiv.org/pdf/2501.02727.pdf
6 Arxiv, Retrieval Augmented Thought Process for Private Data Handling, (Arxiv)https://arxiv.org/html/2402.07812v2
7 Arxiv, Hybrid RAG-empowered Multi-modal LLM for Secure Data Management in Internet of Medical Things, (Arxiv)https://arxiv.org/abs/2407.00978
8 Arxiv, MedRAG: Enhancing Retrieval-augmented Generation with Knowledge Graph-Elicited Reasoning for Healthcare Copilot, (Arxiv)https://arxiv.org/html/2502.04413v1
9 NVIDIA, Evaluating Medical RAG with NVIDIA AI Endpoints and Ragas, (NVIDIA)https://developer.nvidia.com/blog/evaluating-medical-rag-with-nvidia-ai-endpoints-and-ragas/
10 Thiyagarajan, Revolutionizing Healthcare with Retrieval-Augmented Generation, (Radical Data Science)https://radicaldatascience.wordpress.com/2025/02/18/revolutionizing-healthcare-with-retrieval-augmented-generation-rag-for-intelligent-clinical-decision-support/
Generative AI in Upstream Natural Gas: Shell's Exploration Initiative
How Shell partnered with SparkCognition to deploy generative AI for seismic data analysis, reducing exploration time from 9 months to 9 days while achieving significant cost savings and improved drilling success rates.
Optimizing Manufacturing with AI-Driven Multi-Agent Systems
This case study explores how AI-driven multi-agent systems are transforming manufacturing by enhancing inventory management, demand forecasting, and logistics. These solutions leverage AI for predictive analytics, real-time optimization, and intelligent orchestration, driving efficiency and reducing operational costs.