Case Study: Generative AI in Upstream Natural Gas – Shell’s Exploration Initiative
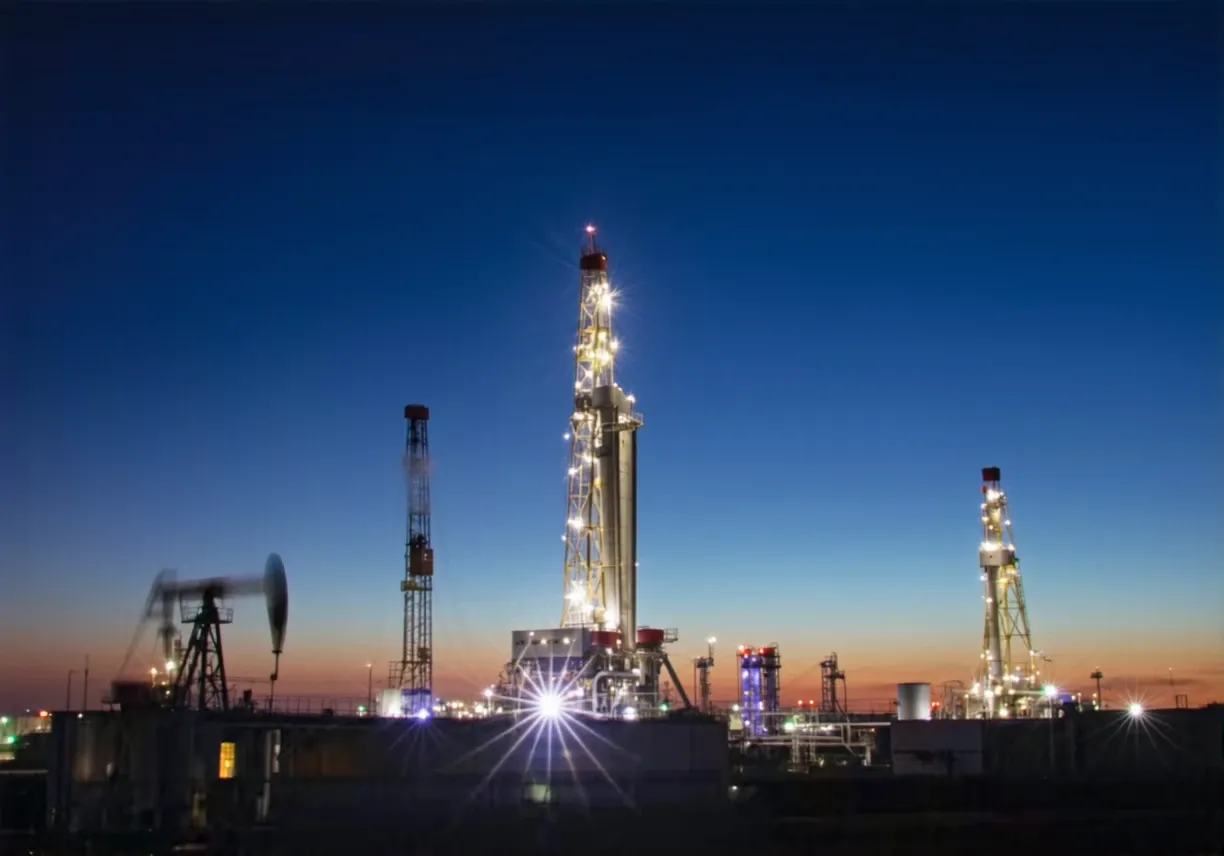
Background
Shell, one of the world’s largest oil and gas producers, recently partnered with AI firm SparkCognition to deploy generative AI in its upstream exploration operations. The collaboration, announced in 2023, focuses on using deep-learning models to analyze seismic data far faster and more efficiently than traditional methods (Shell to use new AI technology in deep sea oil exploration | Reuters). By generating subsurface geological images with minimal input data, Shell’s goal was to accelerate the discovery of new natural gas (and oil) reserves, improve decision-making in drilling, and integrate AI into its broader strategy for safer, more efficient exploration (The Digital Drill: How big oil is using AI to speed up fossil fuel extraction | Global Witness). This case study examines the economic impact and return on investment (ROI) of Shell’s generative AI project, including the key benefits achieved, the cost-vs-savings analysis, challenges faced during implementation, and the long-term outlook for upstream operators embracing GenAI.
Key Economic Benefits and Impact
(The Digital Drill: How big oil is using AI to speed up fossil fuel extraction | Global Witness) Generative AI can transform upstream operations by enabling faster, data-driven decision-making in exploration and drilling.
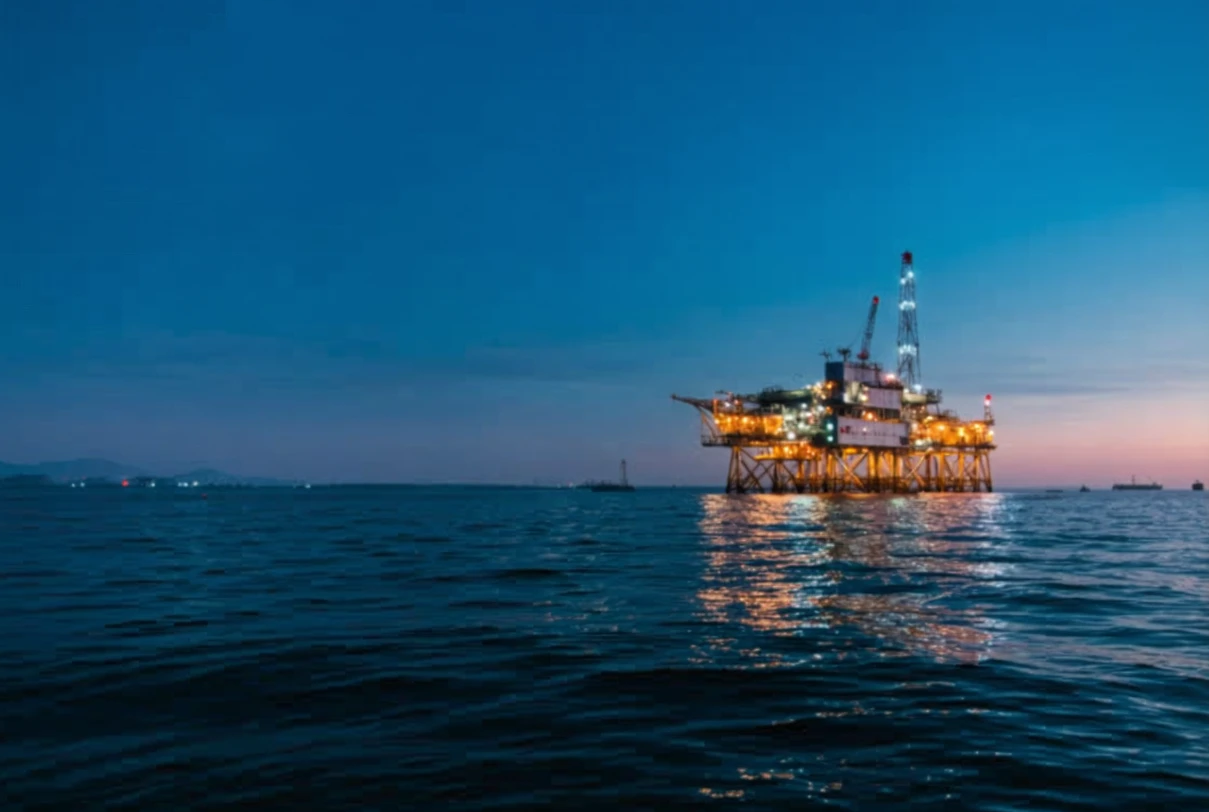
Shell’s deployment of generative AI in exploration has yielded significant economic benefits:
- Dramatically Faster Exploration: The AI-driven workflow can shorten the seismic analysis phase from around nine months to just nine days (Shell to use new AI technology in deep sea oil exploration | Reuters). This ~97% reduction in cycle time accelerates field development decisions and brings new gas production online sooner, boosting the project’s net present value.
- Cost Savings in Data Acquisition and Processing: By using generative models, Shell’s system produces reliable subsurface images with as little as 1% of the seismic data normally required (Shell accelerates deepsea exploration with AI | Offshore). Fewer seismic surveys translate to substantially lower exploration spend – expensive offshore survey campaigns and high-performance computing costs are reduced by on the order of 99% in field trials (Shell accelerates deepsea exploration with AI | Offshore) (Shell to use new AI technology in deep sea oil exploration | Reuters).
- Improved Efficiency and Higher Success Rates: Automating seismic interpretation with AI increases the chance of drilling successful wells. Shell’s partner SparkCognition reports that the generative AI approach has improved exploration efficiency and success, potentially leading to increased production from discovered resources (Shell accelerates deepsea exploration with AI | Offshore). In practical terms, better identification of drill targets means fewer dry holes and more gas recovered per dollar invested in drilling.
- Operational Risk Reduction: AI-driven analysis also lowers risk by providing better subsurface insight upfront. By identifying geologic hazards or poor prospects before drilling, Shell can avoid costly mistakes. (For example, industry use of AI has reduced equipment failure incidents – Shell has reported 40% fewer equipment failures after adopting predictive analytics in operations (AI in Oil and Gas: Preventing Equipment Failures Before They Cost Millions - Energies Media), illustrating how AI can mitigate operational risks.)
Collectively, these benefits improve the economics of upstream projects. Shell’s generative AI expedites the exploration process and trims unnecessary costs, while increasing the volume of gas that can be profitably extracted and reducing the likelihood of failures or delays.
ROI Breakdown – Investment vs Savings
Shell’s case demonstrates a compelling ROI for generative AI in upstream gas operations. The return on investment can be broken down as follows:
- Upfront Investment Costs: Implementing GenAI required investing in data infrastructure, model development, and partnerships. For instance, BP (another energy major) invested about $5 million in a subsurface AI platform to augment its exploration workflow (AI in Oil and Gas: Preventing Equipment Failures Before They Cost Millions - Energies Media). Shell’s collaboration with SparkCognition similarly involved funding AI R&D and deploying new software. These upfront costs (often in the single-digit millions) cover cloud computing resources, AI software licenses, and training staff to use the new tools.
- Operational Savings: The savings from GenAI implementation are significant and ongoing. By slashing seismic survey requirements and computation time, Shell’s approach avoids the cost of extensive fieldwork and processing – potentially saving tens of millions of dollars per project in seismic data acquisition and interpretation. Moreover, optimizing drilling targets yields direct savings; BP’s AI platform “Sandy” now saves it about $10 million every year in operating costs through better planning and asset optimization (AI in Oil and Gas: Preventing Equipment Failures Before They Cost Millions - Energies Media). Shell likewise expects multi-million dollar annual savings from faster project timelines (bringing revenue online sooner) and from not drilling unproductive wells that AI helped rule out.
- Payback Period: Given the magnitude of savings, the payback period for GenAI investments in upstream operations is very short. In BP’s case, the 10M/year cost savings (AI in Oil and Gas: Preventing Equipment Failures Before They Cost Millions - Energies Media) (AI in Oil and Gas: Preventing Equipment Failures Before They Cost Millions - Energies Media). Shell’s generative AI project is on a similar trajectory – the reduction in exploration lead time alone can save enough in overhead and earlier production revenues to pay back the AI investment within the first 1–2 drilling campaigns. In other words, the project achieves positive ROI in under 12 months of operation, after which the efficiency gains directly improve the bottom line.
- Financial Gains and Uplift: Beyond cost savings, GenAI provides a long-term financial uplift by increasing recoverable reserves and production. Faster and more accurate identification of gas deposits means Shell can develop more resources. Industry analysts estimate that AI could boost hydrocarbon recovery factors by 10–20%, effectively increasing proved reserves by a similar margin (AI likely to weigh on oil prices over the next decade, Goldman says | Reuters). More reserves and higher production translate to greater revenue over the field’s life. In Shell’s case, accelerating exploration from 9 months to 9 days also means earlier start-up of production, improving the project’s internal rate of return. These gains come in addition to the direct cost cuts, making the ROI not just about cost reduction but also about higher throughput and revenue. (Notably, oil & gas companies adopting AI have seen an average 32% annual ROI on their AI investments according to an IBM industry survey ( How AI investors can ride the oil and gas boom | Equifund), highlighting the strong financial returns being realized.)
Overall ROI: Combining the above factors, Shell’s GenAI initiative in exploration yields a robust return. The one-time implementation costs are dwarfed by recurring savings (in exploration spend and operating efficiency) and by the increased value of additional gas produced. The project quickly turned profitable and continues to deliver ongoing ROI through lower operating costs and higher output. This validates the business case for generative AI in upstream operations – even conservative estimates show double-digit percentage ROI, and real-world deployments like Shell’s and BP’s have achieved payback in a matter of months and millions in net gains (AI in Oil and Gas: Preventing Equipment Failures Before They Cost Millions - Energies Media) ( How AI investors can ride the oil and gas boom | Equifund).
Implementation Challenges and Solutions
Deploying generative AI in a complex upstream environment was not without challenges. Shell and its partners encountered several hurdles and developed strategies to overcome them:
- Data Quality and Availability: Successful AI models require large volumes of high-quality data, yet upstream data (seismic readings, well logs, etc.) can be noisy, siloed, or incomplete. In fact, studies show poor data quality causes 80% of AI project failures in industrial settings (AI in Oil and Gas: Preventing Equipment Failures Before They Cost Millions - Energies Media). Shell addressed this by investing in robust data management – cleaning and curating historical seismic datasets and integrating data from various sources. They implemented strict data validation and normalization processes to prevent the AI from being misled by bad data. This foundation was crucial to train the generative model effectively and avoid “hallucinations” or errors.
- Legacy Systems Integration: Oil and gas companies often run on legacy software and fragmented databases. Shell faced the challenge of plugging a cutting-edge AI system into its existing exploration workflow and IT infrastructure. Legacy data formats and siloed geological databases initially posed integration roadblocks (AI in Oil and Gas: Preventing Equipment Failures Before They Cost Millions - Energies Media). The solution was to use middleware and cloud platforms to connect disparate systems. Shell leveraged modern data integration tools to pull together seismic data, well reports, and interpretations into a unified platform that the GenAI could access (AI in Oil and Gas: Preventing Equipment Failures Before They Cost Millions - Energies Media). By creating this digital backbone (with adequate data pipelines and APIs), the AI system could be deployed without overhauling all legacy systems, thus smoothing adoption.
- Skill and Knowledge Gaps: Implementing AI required new expertise that Shell’s traditional geoscience teams initially lacked. There was a skills gap, as only a limited number of employees were familiar with machine learning techniques, and 29% of executives cite their core team’s knowledge gap as a barrier to AI adoption (AI in Oil and Gas: Preventing Equipment Failures Before They Cost Millions - Energies Media). In addition, there was cultural resistance – seasoned geologists and engineers were initially skeptical of AI recommendations, preferring established methods ( How AI investors can ride the oil and gas boom | Equifund). To overcome this, Shell pursued an extensive training and change management program. They upskilled their staff through workshops and collaborations, even partnering with universities and consultants to train geoscientists in data science (AI in Oil and Gas: Preventing Equipment Failures Before They Cost Millions - Energies Media). Domain experts were teamed with data scientists to ensure the AI’s outputs were interpretable and trusted. By demonstrating early pilot successes (e.g. the AI accurately re-imaging a known reservoir), Shell gradually won over internal stakeholders.
- Cybersecurity and Data Governance: Connecting critical operational data to AI systems introduced concerns around security and IP protection. An interconnected AI platform could become a target for cyber attacks or inadvertent data leaks. Shell mitigated this by implementing strict cybersecurity measures – encryption of sensitive subsurface data, access controls, and continuous security audits (AI in Oil and Gas: Preventing Equipment Failures Before They Cost Millions - Energies Media). They also aligned the AI deployment with data governance policies to ensure compliance with regulations and protect proprietary geological information. These steps helped build trust that the GenAI system could be used safely in mission-critical processes.
- Executive Buy-In and Budget: As with any emerging technology, securing management buy-in (and budget allocation) was a challenge initially. The project had to prove its value to justify scaling up. Shell addressed this by clearly quantifying the expected ROI – for example, highlighting that even a single avoided dry well or a faster field appraisal could save or earn the company tens of millions. Early results from trial projects (like the field trial that achieved 99% data reduction with maintained accuracy (Shell accelerates deepsea exploration with AI | Offshore)) provided hard evidence. By demonstrating a clear business case – showcasing efficiency gains and cost savings in simulations – the team won strong executive support, ensuring continued funding and prioritization of the GenAI initiative. Once the financial rationale was evident, leadership became champions of the project, helping to drive organization-wide adoption.
Through these strategies, Shell successfully navigated the implementation challenges. The combination of technical solutions (data cleaning, system integration) and human solutions (training, change management, executive engagement) was key to embedding generative AI into upstream operations. As a result, the company not only deployed the technology but also attained the organizational alignment needed to realize its full economic benefits.
Long-Term Outlook for GenAI in Upstream Operations
The positive results from Shell’s generative AI project reflect a broader industry trend: upstream oil and gas companies are increasingly turning to AI to enhance profitability and efficiency. In the coming years, the financial and operational outlook for companies adopting generative AI in exploration and production is highly promising:
- Widespread Adoption and Competitive Advantage: All of the top 20 global oil and gas producers now have clear AI strategies for their upstream businesses ( How AI investors can ride the oil and gas boom | Equifund). This indicates that what was once experimental is becoming mainstream. Companies that aggressively implement AI (including GenAI for subsurface modeling) are likely to gain a competitive edge through lower costs and higher output. Those who lag may face competitive pressure as their peers produce gas more cheaply and efficiently. An IBM report notes that a vast majority of O&G executives (84%) expect AI to be critical to their success in the next few years ( How AI investors can ride the oil and gas boom | Equifund). Generative AI, in particular, will become a standard tool in geoscience and drilling – analogous to how 3D seismic became standard in prior decades – and will be part of the toolkit for maximizing resource extraction.
- Sustained Efficiency Gains: The long-term operational impact of GenAI is a sustained improvement in efficiency across the project lifecycle. As AI models continue to learn and improve with more data, we can expect even faster turnaround times for exploration analysis (potentially real-time prospect generation) and more optimized drilling programs. Routine decisions – from where to drill next, to how to adjust production in a gas field – could be informed by AI-generated insights, minimizing waste and downtime. Notably, Goldman Sachs forecasts that AI could eventually cut upstream project costs by up to 30% through better logistics and resource allocation (AI likely to weigh on oil prices over the next decade, Goldman says | Reuters). For shale gas and other unconventional operations, such productivity gains would significantly lower the breakeven cost of supply.
- Increased Reserves and Recovery: Generative AI and advanced analytics can unlock more hydrocarbons from the same fields. By identifying bypassed pay zones or optimizing well placement, AI helps tap resources that might otherwise remain unreachable. Analysts project an AI-driven increase in recovery factors by 10–20%, which for low-recovery reservoirs means a substantial jump in economically recoverable gas (AI likely to weigh on oil prices over the next decade, Goldman says | Reuters). In effect, AI is extending the life and output of existing assets – a long-term financial boon. Companies adopting these technologies will likely report growth in their reserve base and improved ultimate recovery from fields, strengthening their asset portfolios.
- Faster Innovation Cycles: The success of GenAI in exploration is paving the way for similar innovations in other upstream areas (drilling automation, production optimization, reservoir management). We expect an accelerated innovation cycle where lessons from one AI application inform others. Shell’s project, for example, is already inspiring applications of generative models to onshore exploration and even other industries (Shell accelerates deepsea exploration with AI | Offshore). This cross-pollination means upstream operators will continuously find new uses for GenAI, driving further incremental gains – a virtuous cycle of innovation leading to better economics.
- Financial Resilience and Strategic Value: In the long run, the integration of AI contributes to making upstream operations more resilient to market fluctuations. Lower operating costs and higher recovery rates give companies a buffer against low gas prices by improving margins. As AI optimizes energy use and streamlines operations, firms also reduce wasteful expenditures. All this improves financial resilience and adds strategic value – AI-enabled companies can respond faster to opportunities (e.g. quickly appraise a new gas discovery) and challenges (e.g. diagnose a production issue). Moreover, some AI-driven efficiencies (like reduced need for physical surveys) also support sustainability goals by lowering the environmental footprint of exploration, aligning with long-term industry shifts toward responsible operations (Shell accelerates deepsea exploration with AI | Offshore).
Outlook: Over the next decade, generative AI is poised to become an indispensable part of upstream natural gas operations. Early adopters like Shell are demonstrating quantifiable gains in speed, cost, and output, which will encourage broader adoption across the industry. Financially, we can expect AI-enabled operators to consistently achieve higher ROI on their projects – a trend already observed, as AI deployments have been yielding strong returns in pilot projects ( How AI investors can ride the oil and gas boom | Equifund). As the technology matures, the ROI could improve further, since development costs may decrease while benefits accumulate year over year.
In summary, the long-term outlook is that companies embedding generative AI into exploration, drilling, and production will enjoy lower costs, increased gas recovery, and enhanced operational agility. These improvements translate into superior financial performance (higher profits and asset values) and better risk management. In an industry known for high capital stakes and uncertainty, generative AI offers a way to tilt the odds in the operator’s favor – an investment in efficiency that is already proving its worth and is set to redefine upstream economics in the years ahead.
Sources: The information and data points in this case study are drawn from industry reports, project announcements, and expert analyses, including Reuters, Offshore Magazine, Goldman Sachs research, IBM’s oil & gas survey, and published case studies of AI deployments in the energy sector (Shell to use new AI technology in deep sea oil exploration | Reuters) (Shell accelerates deepsea exploration with AI | Offshore) (AI in Oil and Gas: Preventing Equipment Failures Before They Cost Millions - Energies Media) (AI likely to weigh on oil prices over the next decade, Goldman says | Reuters). These sources provide quantitative evidence of the impact of AI in upstream operations and validate the ROI figures and benefits discussed.