AI Teams: How Multiple AI Agents Are Working Together
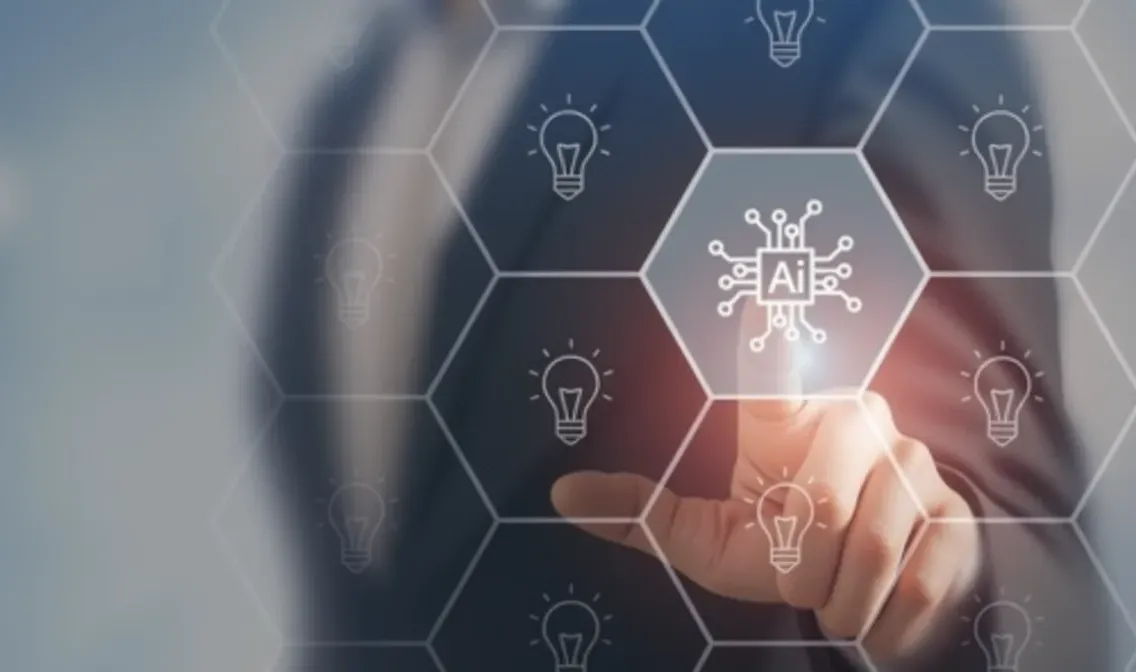
From Solo Stars to Dream Teams
Remember when we thought the key to better AI was just making one really smart model? That's changing fast. Today's exciting trend is getting different AI systems to work together as a team - just like humans do in the workplace.
Why AI Teams Work Better
Imagine a doctor's office where one person had to be the receptionist, nurse, doctor, and specialist all at once. That's what we've been asking single AI models to do. The new approach is much more practical.
When AI systems work as a team, they each get to focus on what they do best. One might be great at understanding images, while another excels at writing, and a third keeps track of facts. Together, they catch each other's mistakes and build on each other's ideas.
These AI teams can actually check each other's work. When one AI makes a mistake or isn't sure about something, another team member can step in to help, just like colleagues reviewing each other's work.
Real Examples Anyone Can Understand
This isn't just a cool idea - it's already happening in the real world:
In hospitals, AI teams are helping doctors spot rare conditions. One AI looks at X-rays, another reviews lab results, and a third checks medical history. Working together, they're helping identify unusual diseases 42% more often than before.
Banks are using AI teams to spot fraud while reducing false alarms. Instead of one system trying to do everything, different AI agents analyze spending patterns, location data, and account history separately before coming together with a final recommendation.
Researchers who once spent months reading thousands of scientific papers can now get help from AI teams that divide and conquer the work. One AI summarizes findings, another connects related studies, and a third identifies trends - turning months of work into days.
How These AI Teams Are Built
Think of AI teams like a well-run project group. There's usually a manager AI that assigns tasks and keeps everyone on track. Specialist AIs handle specific parts of the problem, while a "quality control" AI reviews the work. The whole system remembers what happened before so conversations can build on previous insights.
Real-World Challenges
Building effective AI teams isn't easy. Running multiple AI systems at once requires more computing power and electricity. Getting the AIs to communicate efficiently is tricky - too many messages back and forth slows everything down. And perhaps most challenging: making sure all team members are working toward the same goal without working at cross-purposes.
Looking Forward
For companies interested in this approach, start by identifying specific tasks where having multiple perspectives would be valuable. Think about how the AI team members should communicate and make decisions together. And always keep humans in the loop to provide guidance and oversight.
The Future Is Collaborative
As we move forward, the most powerful AI systems won't be lone geniuses but well-orchestrated teams. The big question isn't whether AI teams will replace single AIs, but figuring out which problems benefit most from a team approach.
The AI That Rewrites Itself: MIT's Breakthrough in Self-Adapting Language Models
Discover how MIT researchers created SEAL, an AI framework that generates its own training data and adapts autonomously, marking a revolutionary step toward truly intelligent machines.
In-Depth Analysis of Model Context Protocol (MCP) Security Practices
A comprehensive examination of MCP security architecture, vulnerabilities, and best practices for organizations implementing AI integrations. Learn about tool poisoning attacks, lifecycle security risks, and mitigation strategies in this detailed analysis.